The rise of artificial intelligence (AI) and data analytics in clinical decision support is helping clinicians understand broader data sets in less time. In this post, you’ll find out where and how these tools are being used, who’s having success, and what new applications are on the horizon.
Who needs these tools?
The short answer is clinicians. The amount of data being generated has now outstripped the time and resources available to review it. A Consensus Study Report titled, Best Care at Lower Cost: The Path to Continuously Learning Health Care in America (The National Academies Press; 2013) cites a 2009 study which found that a physician following accepted guidelines would need over 21 hours a day to meet a standard patient’s acute, preventative, and chronic disease management needs.At a minimum, a clinician seeing a patient reviews the patient’s chart and medical data. If treatment advice is being given, the clinician must select tests. If a drug is being prescribed, the clinician must review an ever-expanding array of drug data to evaluate drug-to-drug interactions. Having AI and analytics tools to manage this enormous amount of data in real time is helping clinicians make better-informed decisions in less time.
How are AI and analytics tools being used?
These tools are being used to offload certain information that was formerly memorized by clinicians, such as drug interactions and treatment protocols. In the days when there were fewer options to treat a specific condition, memorizing drug interactions was fairly easy for a clinician. But with more and more drugs being developed, the complexity is overwhelming, and the potential for human error increases.Using analytic tools, a clinician can type in a drug name and know instantly whether that drug will be effective or potentially cause an adverse reaction in the patient. This ability to “test” drugs in real time means that a clinician can send a patient home with the correct prescription for that patient’s condition.Part of the effort to offload basic reference information is coming from hospitals. They want to improve patient care and limit their liability for adverse drug interactions. Three ways hospitals do this are by reminding overburdened clinicians of things they need to do, providing recommendations when clinicians are unsure of what to do, and correcting errors that clinicians may have made. If a clinician’s treatment is ineffective, the patient has to come back to see the clinician for another prescription and run through several cycles of interaction with that clinician or a new one. AI is reducing these complications and interactions by helping clinicians treat patients effectively the first time they’re seen.
Who’s having success?
In the health technology market, two types of organizations are gaining traction with AI and analytic tools in clinical decision support. The first type already is leveraging an existing data set. One example is Wolters Kluwer, an information services company that traces its roots to publishing. Another is Elsevier, a publisher of medical journals. Both organizations have curated and indexed existing data sets to make them AI-friendly in clinical decision support.The second type of organization is more value driven. Rather than offering a large, curated database, these organizations are helping clinicians with prescriptions, patient advising, and treatment outcomes. For a patient who has a particular diagnosis or a biomarker, AI and analytics tools can help determine an appropriate treatment protocol. That could mean suggesting a medication, like Tarceva, as part of an oncology treatment or, in the case of a pathogen, outlining a sepsis management protocol. Earlier treatment can improve outcomes and reduce the time spent waiting for a clinician to review lab results and treatment options.
What are the short- and long-term outlooks?
In the short term, AI and analytics tools will expand to different therapeutic areas and be used increasingly for treatment and diagnosis. They’re common in oncology and infectious disease management and will likely become more common in cardiovascular care and central nervous disease treatment.Over the next 10 to 20 years, look for AI and analytics tools to be implemented much like auto-pilot technology. Even though auto-pilot technology can launch, fly, and land a plane, pilots and passengers still prefer a human at the controls. With AI, there’s a similar reluctance from both clinicians and patients to the idea of a robot autonomously deciding upon and dispensing patient care. Regulatory agencies agree. For the foreseeable future, AI and analytics tools will see increasing use, but any treatment and diagnostic recommendations will still require a clinician’s approval.
What’s on the horizon?
There’s an industry effort to go beyond structured data sets—that is, standard text and numerical data—and into real-world data. Two big pieces of real-world data are imaging and physician notes. Even though both contain a lot of rich medical data, they remain out of reach for many clinicians because they’re harder to access and interpret than structured data sets.For example, let’s say a patient has a cardiovascular event that’s complicated by an infectious disease. If the infectious disease symptoms appear in the physician notes but not in the general medical record, a new clinician treating this patient will likely miss the symptoms. AI is able to pick up the symptoms from the physician notes and flag the possibility of an infection.Using AI and data analytics tools to synthesize broader data sets isn’t just a passing healthcare technology trend. It’s giving clinicians a more complete picture of a patient than they’ve ever had before. These futuristic tools are facilitating a return to more traditional care, where clinicians can spend time with their patients, rather than poring over an increasing amount of data. Making better-informed decisions in less time can raise the standard of healthcare by making it more efficient to dispense.
Looking for strategic insights for the health technology market? Contact the DeciBio team today to get health technology market research and insights tailored to your most pressing questions.
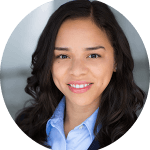
Author | Melissa Campos
Linkedin | campos@decibio.comMelissa Campos is a Senior Associate with extensive experience in development and commercialization of healthcare data analytics platforms and clinical decision support systems. Connect with her on LinkedIn.Contact Melissa and the DeciBio team to get the insights you need to accelerate innovation in your market.Disclaimer: Companies listed above may be DeciBio Consulting clients and/or customers